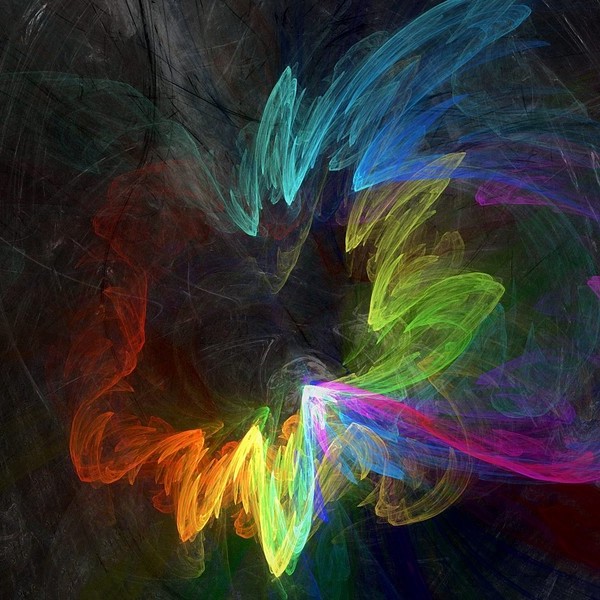
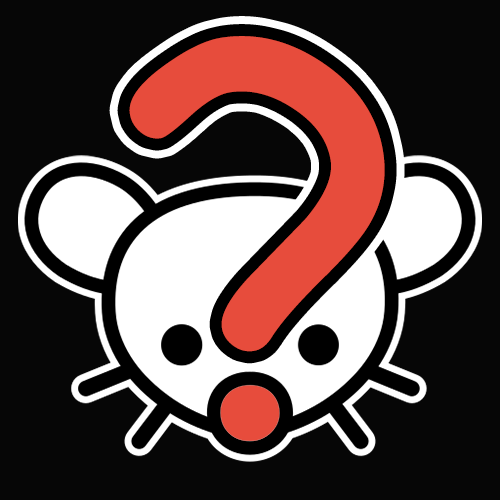
Do you think electing Trump will be read as “wow, the US is taking a principled stance on Palestinian rights” by the world?
Do you think electing Trump will be read as “wow, the US is taking a principled stance on Palestinian rights” by the world?
That’s not how it works at all. If it were as easy as adding a line of code that says “check for integrity” they would’ve done that already. Fundamentally, the way these models all work is you give them some text and they try to guess the next word. It’s ultra autocomplete. If you feed it “I’m going to the grocery store to get some” then it’ll respond “food: 32%, bread: 15%, milk: 13%” and so on.
They get these results by crunching a ton of numbers, and those numbers, called a model, were tuned by training. During training, they collect every scrap of human text they can get their hands on, feed bits of it to the model, then see what the model guesses. They compare the model’s guess to the actual text, tweak the numbers slightly to make the model more likely to give the right answer and less likely to give the wrong answers, then do it again with more text. The tweaking is an automated process, just feeding the model as much text as possible, until eventually it gets shockingly good at predicting. When training is done, the numbers stop getting tweaked, and it will give the same answer to the same prompt every time.
Once you have the model, you can use it to generate responses. Feed it something like “Question: why is the sky blue? Answer:” and if the model has gotten even remotely good at its job of predicting words, the next word should be the start of an answer to the question. Maybe the top prediction is “The”. Well, that’s not much, but you can tack one of the model’s predicted words to the end and do it again. “Question: why is the sky blue? Answer: The” and see what it predicts. Keep repeating until you decide you have enough words, or maybe you’ve trained the model to also be able to predict “end of response” and use that to decide when to stop. You can play with this process, for example, making it more or less random. If you always take the top prediction you’ll get perfectly consistent answers to the same prompt every time, but they’ll be predictable and boring. You can instead pick based on the probabilities you get back from the model and get more variety. You can “increase the temperature” of that and intentionally choose unlikely answers more often than the model expects, which will make the response more varied but will eventually devolve into nonsense if you crank it up too high. Etc, etc. That’s why even though the model is unchanging and gives the same word probabilities to the same input, you can get different answers in the text it gives back.
Note that there’s nothing in here about accuracy, or sources, or thinking, or hallucinations, anything. The model doesn’t know whether it’s saying things that are real or fiction. It’s literally a gigantic unchanging matrix of numbers. It’s not even really “saying” things at all. It’s just tossing out possible words, something else is picking from that list, and then the result is being fed back in for more words. To be clear, it’s really good at this job, and can do some eerily human things, like mixing two concepts together, in a way that computers have never been able to do before. But it was never trained to reason, it wasn’t trained to recognize that it’s saying something untrue, or that it has little knowledge of a subject, or that it is saying something dangerous. It was trained to predict words.
At best, what they do with these things is prepend your questions with instructions, trying to guide the model to respond a certain way. So you’ll type in “how do I make my own fireworks?” but the model will be given “You are a chatbot AI. You are polite and helpful, but you do not give dangerous advice. The user’s question is: how do I make my own fireworks? Your answer:” and hopefully the instructions make the most likely answer something like “that’s dangerous, I’m not discussing it.” It’s still not really thinking, though.
This, and see also “minmaxing,” the process of optimizing something (usually your character in a game) to get minimum penalty and/or maximum benefit, usually ignoring anything like realism or storytelling and focusing entirely on the stats and numbers.
No. The headsets are disabled when the play starts or when the play clock goes below 15 seconds.
These models aren’t great at tasks that require precision and analytical thinking. They’re trained on a fairly simple task, “if I give you some text, guess what the next bit of text is.” Sounds simple, but it’s incredibly powerful. Imagine if you could correctly guess the next bit of text for the sentence “The answer to the ultimate question of life, the universe, and everything is” or “The solution to the problems in the Middle East is”.
Recently, we’ve been seeing shockingly good results from models that do this task. They can synthesize unrelated subjects, and hold coherent conversations that sound very human. However, despite doing some things that up until recently only humans could do, they still aren’t at human-level intelligence. Humans read and write by taking in words, converting them into rich mental concepts, applying thoughts, feelings, and reasoning to them, and then converting the resulting concepts back into words to communicate with others. LLMs arguably might be doing some of this too, but they’re evaluated solely on words and therefore much more of their “thought process” is based on “what words are likely to come next” and not “is this concept being applied correctly” or “is this factual information”. Humans have much, much greater capacity than these models, and we live complex lives that act as an incredibly comprehensive training process. These models are small and trained very narrowly in comparison. Their excellent mimicry gives the illusion of a similarly rich inner life, but it’s mostly imitation.
All that comes down to the fact that these models aren’t great at complex reasoning and precise details. They’re just not trained for it. They got through “life” by picking plausible words and that’s mostly what they’ll continue to do. For writing a novel or poem, that’s good enough, but math and physics are more rigorous than that. They do seem to be able to handle code snippets now, mostly, which is progress, but in general this isn’t something that you can be completely confident in them doing correctly. They make silly mistakes because they aren’t really thinking it through. To them, there isn’t really much difference between answers like “that date is 7 days after Christmas” and “that date is 12 days after Christmas.” Which one it thinks is more correct is based on things it has seen, not necessarily an explicit counting process. You can also see this in things like that case where someone tried to use it to write a legal brief, where it came up with citations that seemed plausible but were in fact completely made up. It wasn’t trained on accurate citations, it was trained on words.
They also have a bad habit of sounding confident no matter what they’re saying, which makes it hard to use them for things you can’t check yourself. Anything they say could be right/accurate/good/not plagiarized, but the model won’t have a good sense of that, and if you don’t know either, you’re opening yourself up to risk of being misled.
Protect me from knowing what I don’t need to know. Protect me from even knowing that there are things to know that I don’t know. Protect me from knowing that I decided not to know about the things that I decided not to know about. Amen.
Lord, lord, lord. Protect me from the consequences of the above prayer. Amen.
That’s part of the point, you aren’t necessarily supposed to have an empty mind the whole time. I mean, if you can do that, great, but you aren’t failing if that’s not the case.
Imagine that your thoughts are buses, and your job is to sit at the bus stop and not get on any of them. Just notice them and let them go by. Like a bus stop, you don’t really control what comes by, but you do control which ones you get on board and follow. If you notice that you’ve gotten on a bus, that’s fine, just get off of it and go back to watching. Interesting things can happen if you just watch and notice which thoughts go by, and it’s good practice for noticing what you’re thinking and where you’re going and taking control of it yourself when it’s somewhere you don’t want to go.
This is the key with all the machine learning stuff going on right now. The robot will create something, but none of them have a firm understanding of right, wrong, truth, lies, reality, or fiction. You have to be able to evaluate its output because you have no idea if the robot’s telling the truth or not at that moment. Images are pretty immune to this because everyone can evaluate a picture for correctness or realism, and even if it’s a misleading photorealistic image, well, we’ve already had Photoshops for a long time. With text, you always have to keep in mind that the robot might be low quality or outright wrong, and if you aren’t equipped to evaluate its answers for that, you shouldn’t be using it.
The doom and gloom predictions have always been about slow but inexorable changes in the climate. Not that suddenly a mega hurricane is going to rip Florida out of the ground and toss it into the ocean, but that weather is going to get worse and more extreme, that sea levels will rise, and more and more places will gradually become uninhabitable as conditions get worse. There won’t be single things that you can point to and say “that one was global warming”, it’s about trends that are harmful for us in the long term. If you eat a chocolate bar’s worth more calories than you burn every day, it sounds like doom and gloom to say you’ll gain 200 pounds if you don’t change anything, and you won’t be able to point to any one meal as something to be concerned about because that’s not really out of the ordinary for a day… but slowly and steadily, you’ll gain weight, and if nothing changes you will get there eventually.
And even though you aren’t owed dramatic destruction, and shouldn’t require it to believe the thousands of people who study this as their life’s work and all agree that things are dire and not getting better fast enough… you’ve literally just lived through the hottest twenty or so days in recorded history. Is that a coincidence, do you think?
Archive Team looked at this about 10 years ago and found it basically impossible. It was around 14 petabytes of information to fetch, organize, and distribute at the time.
https://wiki.archiveteam.org/index.php/INTERNETARCHIVE.BAK